Population Research Discovery Seminars
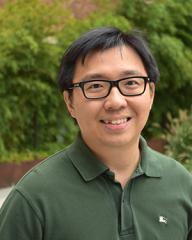
Population causal inference: old wine in a new bottle?
Gary Chan, Associate Professor of Biostatistics and Health Services, UW
02/23/2018
12:30-1:30 PM PT
The quest of causal inference using non-experimental data has been a long-standing one. While causal interpretation is often limited by strong assumptions, there has been a growing interest among statisticians to develop new methodologies for inferring population causal parameters, which is often defined with the aid of counterfactual outcomes. Some basic concepts and assumptions will be presented, followed by a discussion of recently developed nonparametric covariate balancing methods. Connection of covariate balancing to a century-old concept of standardization is given. Gary will use real data examples to illustrate that various statistical methods can lead to different conclusions, and argue that the result from covariate balancing method is more likely to be similar to that of a randomized experiment.
Gary Chan is interested in developing statistical methodologies that are simple to implement, easy to understand and enjoy improved/optimal statistical properties. Although it seems impossible to achieve all these aims simultaneously for most problems, Gary thinks they are necessary conditions for statistical methods to be practically useful. Gary has very broad research interests, including survival analysis, missing data/causal inference, complex sampling designs and general semiparametric and nonparametric methods.