CSDE Trainees Seminars
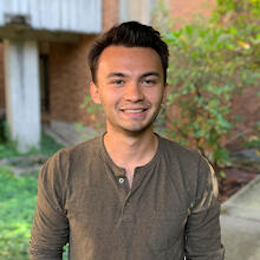
Predicting Individual-Level Mortality with Traditional and Machine Learning Methods
Nicholas Irons, PhD Student, Department of Statistics, UW
Register HERE.
01/06/2023
12:30-1:30 PM PT
101 Hans Rosling Center
Individual-level mortality prediction is a fundamental challenge with implications for individuals and societies, enabling life planning, targeting of high-risk individuals, and organization of social interventions. Demographers have been primarily concerned with mortality analyses at a macro level, leveraging strong regularities in mortality rates. Besides clinical settings, individual-level mortality predictions have been largely overlooked. We use the US Health and Retirement Study, a representative survey of people over 50, and estimate 12 statistical and machine learning models using over 100 predictors. Machine learning and traditional models report comparable accuracy and relatively high predictive and discriminative performance, particularly when including time-varying information (best integrated Brier Score 0.110 and mean Area Under the Curve 0.874). We observed consistent inequalities in lifespan predictability, with predictions for men, people of color, and low-educated respondents being less accurate than for their respective counterparts. Finally, we see minimal variation in the top features across groups, with age, diabetes, and smoking behaviors relevant predictors.
Nicholas Irons is a PhD Student in the Department of Statistics at the University of Washington. His research focuses on development of methods and applications of (Bayesian) statistics to the social sciences, with the goal of informing public policy design and evaluation. He is advised by Adrian Raftery and his work is supported by an NICHD training grant to the UW Center for Studies in Demography & Ecology.